Artificial intelligence (AI) is the hottest trend to hit automotive assembly lines since lean manufacturing in the 1990s. Automakers and suppliers around the world are scrambling to adopt AI technology to boost efficiency, improve quality and reduce errors in their factories.
Automotive AI applications include next-generation vision systems for adhesive dispensing, screwdriving and welding applications, plus parts inspection, plant layout and predictive maintenance. The technology is also making conveyors, robots, vision systems and other production tools more intelligent.
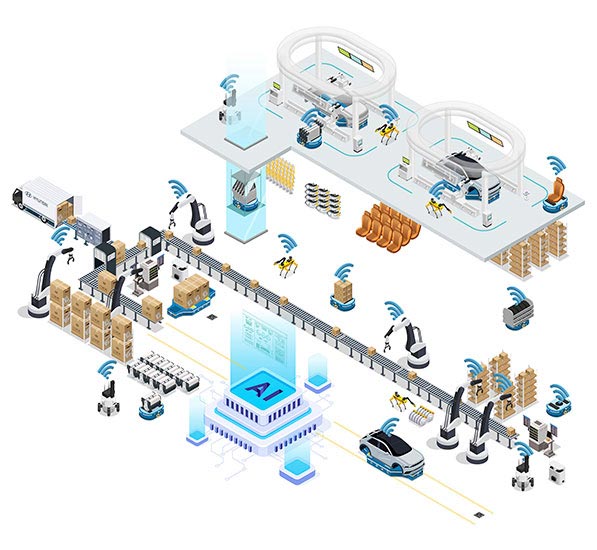
Artificial intelligence is making conveyors, robots, vision systems and other production tools more intelligent. Illustration courtesy Hyundai Motor Group
“Improving the quality of vehicles and simultaneously improving margins have always been the goals for automotive OEMs,” says Ravi Kunju, chief product and strategy officer at software firm Altair Engineering Inc. “Every technology in use today is working toward achieving just this.
“AI helps accelerate these efforts from design to manufacturing in a continuous loop that we define as ‘One Total Twin,’” explains Kunju. “This leverages synthetic data from computational sciences and raw data from the factory floor, converging them with data sciences, such as AI and machine learning, to create a unique value.
“The implications from digital twin technology are substantial at every stage of vehicle design and manufacturing,” notes Kunju. “While [this] is not new and has been in use for some time, the advent of ChatGPT has made AI a popular topic.
“The biggest benefit of using AI in car manufacturing is that businesses are asking themselves what they can learn from the entire digital data infrastructure within their organization,” Kunju points out. “The implications are massive.”
Quality Control
According to Kunju, AI is having a dramatic impact on automotive manufacturing when it comes to quality control and predictive maintenance applications.
“A variety of conditions can affect manufacturing, resulting in variability and inconsistencies,” says Kunju. “AI is increasingly used to predict the right process parameters based on data for what has historically worked or failed.
“Less than 10 percent of factory data from machines is utilized today,” claims Kunju. “Analyzing this data unlocks potential for improvements in process across multiple manufacturing processes.
“Many tasks that are extremely manual , difficult to scale or labor intensive, such as visual inspection of assembly gaps and weld quality, are being trained using photo sensors in combination with advanced machine learning and AI,” explains Kunju. “This can be extended to any other quality criteria, as long as they can be easily scored from a qualitative to a quantitative measure, which requires good understanding of the domain of interest.”
AI has allowed for the use of many more sensors on assembly lines and paved the way for increased data acquisition. The next phase of adopting AI will be to streamline the data and use human intelligence to convert the qualitative data into quantitative metrics that attempt to measure outcomes, achieve reinforced learning and automate production equipment to recorrect on the fly.
“All of this requires a tectonic shift in data-driven decision making and intentional organizational transformation,” warns Kunju. “Some manufacturers will emerge as leaders, taking advantage of AI quickly, while others will need to play catch up.”
“The automotive industry has witnessed a remarkable transformation with the integration of artificial intelligence,” adds Iu Ayala, CEO of Gradient Insight Ltd., an AI consulting firm that specializes in computer vision technology. “One of the most significant trends is the application of computer vision in quality control processes along assembly lines.
“Computer vision algorithms, honed through years of research and development, are now adept at identifying even the minutest defects in car components, ensuring a level of precision that was previously unattainable,” says Ayala.
“Take, for instance, our recent collaboration with a leading automaker,” explains Ayala. “We implemented a computer vision system that analyzes paint quality on car bodies.
“Through advanced image recognition techniques, the system can detect imperfections such as uneven coatings or color discrepancies,” claims Ayala. “This not only ensures a flawless finish, but also significantly reduces the likelihood of defects reaching the end consumer, thereby enhancing overall product quality.
“Another notable trend is the utilization of AI-powered predictive maintenance,” says Ayala. “In a dynamic production environment, unexpected machinery breakdowns can lead to costly downtime.
“By leveraging machine learning algorithms, we’ve assisted car manufacturers in predicting equipment failures before they occur,” notes Ayala. “This proactive approach enables scheduled maintenance, preventing disruptions and optimizing the efficiency of the assembly line.”
Numerous Products
Automotive engineers can choose from a wide variety of AI-enhanced products, including the following:
Coherix Inc. recently developed an inspection system that uses artificial intelligence and machine vision technology to ensure that the correct amount of adhesive is applied during the battery assembly process. The company has also created software capable of automatically adjusting the application of adhesives at line speed to improve quality, cut downtime, and reduce labor and material costs.
Last year, Hexagon’s Manufacturing Intelligence division launched an automated robotic inspection cell called PRESTO that enables manufacturers to reduce quality inspection times, increase efficiency and streamline workflows. It enables end-to-end inspection in a turnkey package and is powered by 3D-laser scanning. That ensures that any surface, even highly reflective materials, can be easily inspected.
The Vaira platform from Pleora Technologies Inc. integrates machine vision cameras, lighting and processing software with AI-based inspection and traceability apps, enabling manufacturers to automate and upgrade error-prone assembly processes.
Micropsi Industries’ MIRAI enables robots to handle variance in shape, position, color and light conditions in the work space. It generates robot movements in real time and can respond automatically to movements of the target object. The system takes control of the first and last centimeters of the robot’s path, eliminating the need for rigid fixturing of parts.
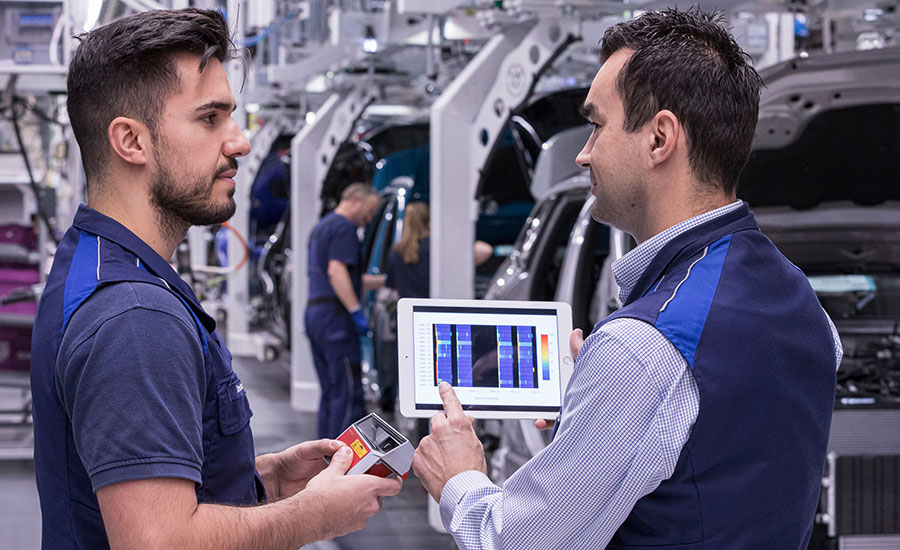
While many workers fear AI could replace their job, AI cannot replace human intelligence. Photo courtesy BMW AG
“Dealing with real-world variance is the largest benefit of using AI for automotive production,” says Matt Jones, vice president of U.S. sales and operations at Micropsi Industries GmbH. “In an ideal world, every part you make, or every process your company does, is identical from day to day. In reality, however, there’s many variables that can come into play, such as shade of color, lighting conditions, positional shifts—even if only a few microns or millimeters—and countless other potential differences.
“When a human works at a station with these variances, our brains barely have to think twice about how to react,” explains Jones. “That just isn’t true for ‘dumb’ simple cameras or robots. That’s where AI can make the needed adjustments, just like a human would."
Micropsi's MIRAI product is being used for a variety of automotive assembly applications, including bin picking, bolt tightening, cable plugging for end-of-line testing and wire harness production. Jones says it has been “a real game changer” for many companies.
“One customer we worked with recently simply needed to put a small black rubber part (let’s call it a bumper) into a black plastic fixture,” he points out. “Normally, this would be quite difficult to do with cameras, as it’s a black-on-black insertion task.
“Also, the bumper and the fixture weren’t in extremely consistent positions or orientations,” adds Jones. “There are a few potential ways to solve this, one being to use force sensing on a robot. When that’s being done, however, the robot will keep trying to find the right location by bumping into the part until it feels the right force (meaning the part can slide into the slot).
“This is both painfully slow and not ideal, as you’d rather not keep bumping the parts together and risk potential damage,” says Jones. “However, with MIRAI, the customer is able to show our system the right technique, and the robot can move quickly and flawlessly to execute the task.”
Micropsi engineers recently teamed up with DEPRAG to develop an automated screwdriving system that can handle variance in manufacturing workstations. It overcomes complexities in screwdriving assembly processes that are extremely hard for most automation systems.
Once fitted with MIRAI, a screwdriving robot can perceive its workspace and correct its movement where needed as it performs a task. Position and angle tolerances, as well as production tolerances, can be compensated on the component.
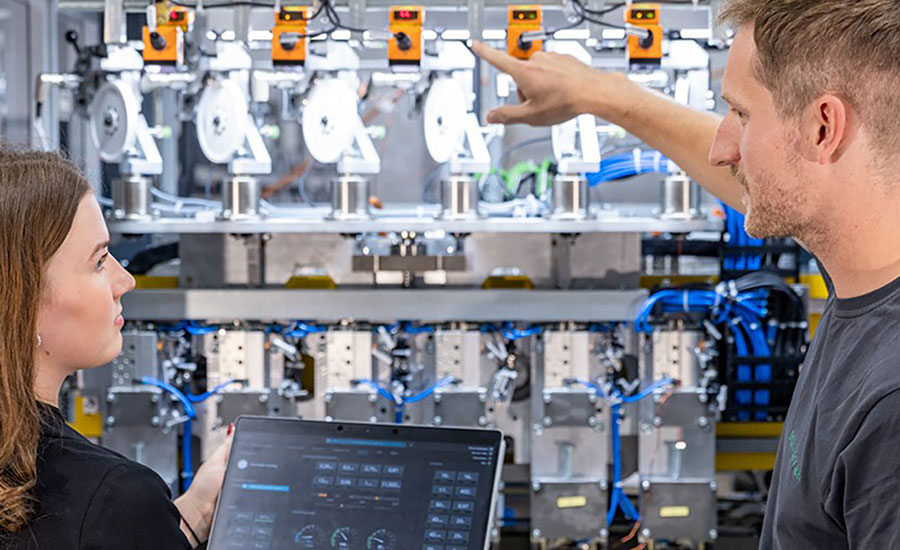
Dispelling misconceptions and offering transparent information about the benefits of AI is important. Photo courtesy Schaeffler Group
Myths and Misunderstandings
Despite the numerous advantages of using artificial intelligence for automotive assembly applications, some engineers are grappling with myths that surround the technology.
“One of the biggest misunderstandings is the general idea that AI can get ‘out of control’ or start having a mind of its own,” says Jones. “While machine learning is extremely impressive and almost feels like these algorithms can be ‘brains,’ the systems are still fed by real-world data.
“Whether that’s images, text or numerical data, they can only work if they have data being fed to them,” explains Jones. “Many companies only use something called supervised learning.” That means the system only improves and changes when the user tells it to.
“Basically, you have learning mode and execution mode,” Jones points out. “This is extra important in manufacturing, because you don’t want the AI to learn bad habits or consider bad data. I think sci-fi movies of the 1980s and 1990s really made AI look a lot crazier and uncontrollable than it really is.”
Another concern involves the ethical use of AI in decision-making processes.
“In autonomous manufacturing environments, AI systems make critical decisions that impact production outcomes,” says Gradient Insight’s Ayala. “Striking the right balance between efficiency and ethical considerations becomes crucial, raising questions about transparency, accountability and the potential biases embedded in AI algorithms.
“The rapid evolution of AI technologies necessitates continuous upskilling of the workforce,” adds Ayala. “Integrating AI into the production line requires skilled personnel who can navigate and optimize these technologies.
“Collaborative efforts between academia, industry and policymakers are vital to establish comprehensive training programs that equip workers with the necessary skills for the AI-driven future of manufacturing,” claims Ayala.
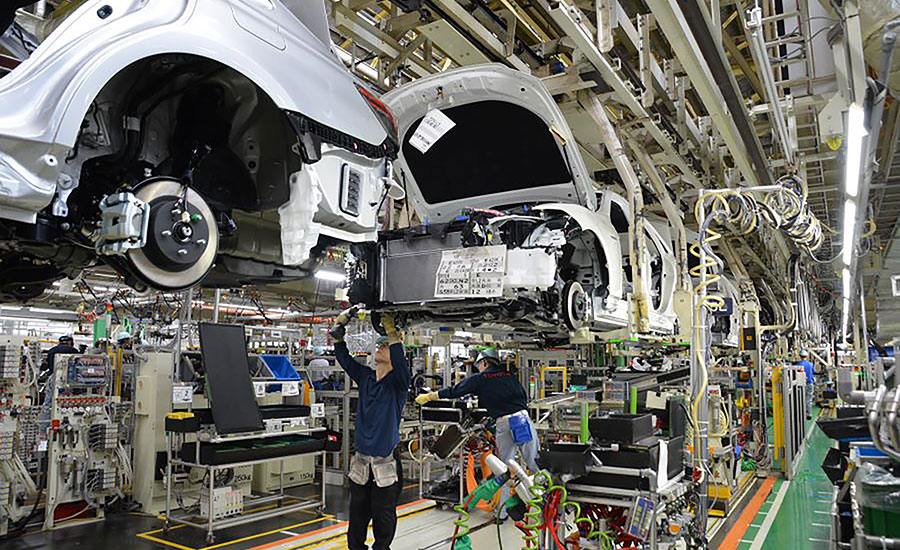
Automakers and suppliers are scrambling to adopt AI technology to boost efficiency, improve quality and reduce errors. Photo courtesy Toyota Motor Corp.
AI in Action
Automakers around the world are deploying cutting-edge AI applications in their factories. One of the companies leading the way is BMW AG.
Its plant in Regensburg, Germany, recently implemented a state-of-the-art system that monitors assembly line conveyors. The predictive maintenance technology identifies potential faults early, avoiding more than 500 minutes of disruption every year and maintaining optimal vehicle production flow.
BMW vehicles, such as the 1 Series, X1 and X2, are attached to mobile load carriers or skid systems that pass through the factory in a chain. The new monitoring system does not require any additional sensors or hardware, but evaluates existing data from installed components and conveyor element control. An alarm sounds if anomalies are found.
The load carriers used to transport vehicles through assembly send various data to the carrier control system. This data is then transmitted via the carrier and plant control system to BMW’s predictive maintenance cloud platform.
An algorithm constantly searches for irregularities, such as fluctuations in power consumption, abnormalities in conveyor movements or bar codes that are not sufficiently legible, which could trigger a malfunction. If anomalies are found, the maintenance control center receives a warning message, which it assigns to the maintenance technician on duty.
“This enables us to respond quickly to any kind of fault report and take the affected vehicle out of the cycle,” says Oliver Mrasek, project manager. “Machine-learning models developed in-house were also implemented in the system, which uses heat maps with various color codes for different abnormalities to visualize the model’s findings. This allows us to map different fault patterns in various components and respond to them in a targeted manner.”
Mrasek and his colleagues are currently in the process of connecting additional installations, optimizing the system and integrating recommended actions into fault messages. They also plan to further exploit the possibilities of artificial intelligence, with the system learning to estimate how much time remains between detection of the fault and the potential stoppage.
“We are currently testing whether we can also use the system for the equipment used to fill our vehicles with brake fluid and coolant,” notes Mrasek.
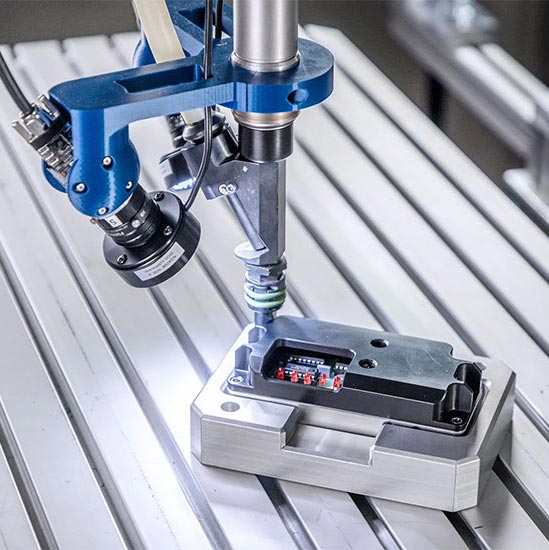
This automated screwdriving system uses AI to handle variance in manufacturing workstations. Photo courtesy Micropsi Industries GmbH
Hyundai Motor Group is harnessing AI at its new Innovation Center Singapore (HMGICS). The seven-story urban facility features a highly automated flexible production system to assemble electric vehicles such as the IONIQ 5 and IONIQ 6.
“[The] cell-based production system leaves behind the traditional conveyor-belt manufacturing approach to achieve unsurpassed standards of flexibility and automation,” says Alpesh Patel, vice president of HMGICS. “Approximately 50 percent of all tasks are carried out by 200 robots, with humans, robotics and AI systems achieving unprecedented levels of collaboration thanks to integration made possible by a digital twin platform.
“Robots perform assembly, inspection and production facility organization, and take care of more than 60 percent of component process management, ordering and transportation,” explains Patel. “This frees humans from repetitive and laborious tasks to focus on more creative and productive duties.
“With the help of robotics, AI, and the Internet of Things, we’ve built a human-centric manufacturing innovation system that can respond to changes in mobility, processes and products with agility and flexibility,” notes Patel.
The facility uses AI-enhanced vehicle inspection cells and automated logistic systems. The later operation begins when delivery vehicles arrive at the loading dock on the first floor. When the cargo from trucks is dropped off on a conveyor belt of the automated logistics system, a robotic arm greets it at the entrance. Then, a robot automatically removes straps and packaging material to reveal the parts inside the box.
AI technology determines the size of components to improve logistical efficiency, by categorizing different storage locations depending on physical size. After this scanning is completed, large parts are moved to the bulk warehouse, while smaller parts are moved to the heavy goods warehouse.
According to Patel, an AI algorithm is linked to a system that can view car orders across the factory, sorting the necessary parts and stacking them into set-box racks in the order in which the vehicles enter the production cell.
“AI comes into play even when parts are moved into production cells and a car begins to be assembled,” says Patel. “[The system identifies] the parts needed for each cell in advance. Then, AI sets the movement path for an autonomous mobile robot so that the parts loaded onto the mobile rack can be transferred to the corresponding cell.”
In addition, AI analyzes supply and demand for specific parts, current stock status and future production plans to automatically order necessary parts.
Other companies bullish on the benefits of AI technology include Daimler Truck North America, a leading producer of heavy-duty commercial vehicles.
“We are currently working on a couple of projects to demonstrate AI image capture and software to learn and identify critical assembly safety processes progressively,” says Joanna Cooper, general manager of Daimler Truck’s flagship factory in Mount Holly, NC.
“AI and digital tools will enable us to control and act on nuances in the end-to-end supply chain,” explains Cooper. “We will be able to stabilize plant operations such that only the trucks that can actually be produced are scheduled, and everything else remains in a holding pattern until these criteria are met.
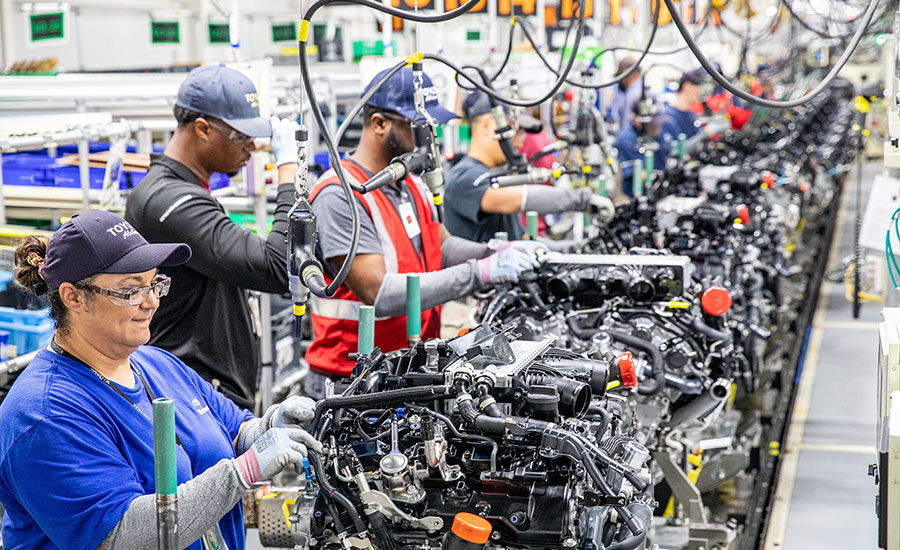
Artificial intelligence is being used for a variety of automotive assembly applications. Photo courtesy Toyota Motor Corp.
Future Anticipation
Artificial intelligence is predicted to grow by leaps and bounds in the future. But, automotive engineers need to be vigilant about the technology’s pros and cons.
“AI is still very new, and governance will be very important,” warns Cooper. “Ethical concerns, biases in algorithms and the risk of misuse are some of the drawbacks to advancing the technology.
“As the technology develops, it will be important for developers, practitioners and users to strike a balance between innovation and responsible deployments,” claims Cooper. “We must also not give in to blind acceptance. Critical thinking will be important to ensure that information integrity is upheld.
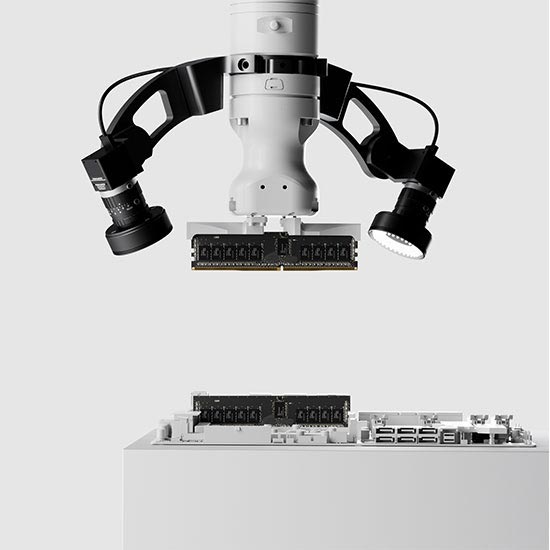
AI can make needed adjustments on an assembly line, just like a human would. Photo courtesy Micropsi Industries GmbH
“One element of change management we often overlook is bringing our team members along with us and explaining the ‘why’ of change,” says Cooper. “The use of AI will enable our team members to perform their work differently. We must explain to them all the ways they will add value in the new landscape and curtail the fears that they will become obsolete.”
“The growing integration of AI within auto manufacturing is poised to transform the very core of the automotive sector and its assembly procedures,” adds Reghu Hariharan, cofounder at Quantiphi Inc., an AI and data analytics software company. “Ranging from heightened automation and anticipatory maintenance, to adaptable production scheduling and enhanced quality control, the advancements we’re seeing, and we’ll continue to see, will play a pivotal role in [creating] a holistic transformation with an eye to enhanced precision, quality and efficiency.
“Fostering continued confidence, dispelling misconceptions and offering transparent information about benefits and protective measures in AI use is essential as [automakers] continue to adopt and utilize AI-driven technologies,” says Hariharan. “By doing so, we can mitigate and guardrail potential lingering concerns and shift focus to effective positioning and use in the automotive industry.”
“Factories of the future will be very different from the factories of today, including changes in both assembly and component-level manufacturing,” predicts Altair’s Kunju. “In the future, we will see more machines and automation, and reduced manpower in factories.
“While there will be less workers, there will be a shift to an increase in knowledge workers who will be the source of providing key domain intelligence,” Kunju points out. “These knowledge workers will constantly improve AI and machine learning models using irreplaceable human intelligence. We will see increased use of digital twin technology at every stage of design and manufacturing, with data being key.
“There is absolutely no AI without organized data and good domain expertise,” warns Kunju. “For AI to be more widely used, we will need to see organizations take data management seriously from design all the way to manufacturing.
“Today, less than 10 percent of the data from machines is processed and probably less than 1 percent of the virtual simulation data is organized and managed properly,” claims Kunju. “This leaves a wide gap in understanding what causes many failures.
“The use of AI also comes with challenges that will require massive scaling of data infrastructure,” adds Kunju. “With it comes issues of data governance and security, as product design and process manufacturing are sacred for automakers and their suppliers.
“While many workers fear AI could replace their jobs, AI cannot replace human intelligence,” says Kunju. “However, the humans that do not embrace AI are the ones that should be concerned about being replaced.”
NOTE: This article was researched and written 100 percent by human – AI was not used in content creation.
Learn about new products for automotive assembly
RU Series/Servo Indexing
Tooling Frames for Automotive Manufacturing